What we’re thinking
Artificial intelligence (AI) and machine learning (ML) are powerful operant resources that may be offered in a proposition’s progress resource mix.
Spohrer usefully reminds us, in “Service in the AI era”, that AI can:
- automate – in the progress economy means replacing an employee either in the seeker organisation or the helper’s resource mix
- augment – enhancing an employee’s skills and knowledge
Technically access to these systems is highly scalable and so they can democratise access to skills and competence previously hard to get (required too high equitable exchange). They are a force multiplier.
progress sought | description |
---|---|
predicting values | predict an output value given some input parameters – e.g. Predictive maintenance, fraud detection, recommendation systems |
classifying data | classify some input parameters into one or more pre-determined classes – e.g. sentiment analysis, image feature detection, object detection |
discovering categories | discover categories within a given a set of data – e.g. customer segmentation |
processing streams | “understand” a given input stream – e.g. natural language processing, examining learning |
translating streams | turn an input stream into a output stream (that contains essentially the same information) – e.g. automatic subtitling, language translation |
generating outputs | generate new content. e.g. generative AI, large language models, autocomplete systems |
Editing / writing from here
Artificial intelligence (AI) and machine learning (ML) are powerful tools that can help progress seekers make progress. They are ripe to be leveraged in progress propositions. Here’s how they look through the progress economy lens.
What is AI? They are systems that encapsulate, usually quite narrow and specific, skills and knowledge. They differentiate themselves from other systems in that they learn those skills and knowledge, rather than having them programmed into them.
And we use AI to achieve some combination of:
- helping progress seeker to make better progress
- helping progress seeker to make existing progress better
- reducing one, or more, of the six hurdles to progress.
As such, they can either automate or augment, as the book “Service in the AI Era” reminds us. In other words, they impact the employee element of progress resource mix. They are offered instead of some or all employees (automating) or they empower offered employees (augmenting).
Generically AI can help with the following classes of functional progress sought:
- predicting values
- classifying data
- discovering categories
- processing streams
- translating streams
- generating streams
Of which there are many examples such as predictive maintenance, fraud detection, image recognition, customer behaviour segmentation, examining learning, customer service agents, virtual assistants, and so on.
Non-functional progress…
Regarding contextual progress, AI
Since these systems are scalable they democratise access to skills and knowledge. Which minimises the lack of resource and service elsewhere progress hurdles. But there are challenges with the remaining four hurdles.
Let’s explore AI through the lens of the progress economy.
It makes sense to first look at AI in terms of what generic progress sought it can help with. Then to see exactly where it fits in the progress resource mix. And finally to consider what we can learn from the six progress hurdles.
progressing using artificial intelligence
Artificial intelligence is a system that encapsulates specific skills and knowledge. It is applied to help a progress seeker attempt progress (moving over time to a more desirable state). And these systems differentiate themselves by learning the skills and knowledge. Rather than having those encoded by a programmer.
Those AI systems with which a progress seeker integrates during progress attempts are a system component of the progress resource mix (one of two parts of a progress proposition).
progress resources mix: the varying amounts (including none) of four, skills and knowledge encapsulating, resources:
- employees,
- systems,
- goods,
- physical resources
offered by the helper for integration with the seeker during progress attempts.
Often the AI will be offered instead of an employee. Although it may be offered instead of a less intelligent system.
Spohrer reminds us, in “Service in the AI era”, that AI in a service can:
- automate – for us meaning take the place of an employee/system, or
- augment – which for us means empowering an employee
The former, automating, is the situation above. Whereas augmenting means empowering an employee.
And lets not forget that AI may be used ”behind the scenes”. Perhaps predictive maintenance on servers, scheduling things, or analysing quality, for example. To explore those in the progress economy just requires a re-calibration of who is the progress seeker. From external to internal. And then all we discuss below is applicable.
In both the automating and augmenting cases,the purpose of the AI is to achieve some combination of*:
- helping progress seeker to make better progress
- helping progress seeker to make existing progress better
- reducing one, or more, of the six progress hurdles.
*this comes from the definition of innovation in the progress economy.
We’ll come back to the last point of reducing hurdles later in this article. But as a short summary, as these systems are scalable they enable the democratisation of access to skills and competence. Which should minimise both the lack of resource and service elsewhere progress hurdles.
As for the first two points about progress, let’s dig a little deeper.
What Progress can AI help with?
We define progress in the progress economy as:
progress: moving over time to a more desirable state
And that desirable state comprises of functional and non-functional elements, informed by context. A sort of “I want to get from one side of London to the other, quickly, during rush hour”. Or ”I want to create an image in the style of Van Gogh but I’m not artistic”. ”I need advice on a topic but I’m busy so need to interact as and wen I can”.
What we’ll do now is explore where AI can best help with these elements of progress. Starting with functional progress.
Functional progress sought
There is an apparant magic behind the AI of today capturing the minds and exciting everyone. At the time of writing, for example, ChatGPT is in headlines everywhere. And I’m sure as you read this that will have been surpassed by other AI systems wowing us.
But behind the magic is an abstract set of progress sought that AI can enable. And this is not to diminish what is being achieved. Rather it is useful to know abd then we can look at the progress our seekers are seeking and see if there’s a match. Stephen Wolfram tells us, for example, that when Chat GPT is writing an essay, what it’s really doing is:
…just asking over and over again “given the text so far, what should the next [reasonable] word be?”—and each time adding a word…having previously scanned millions of pages of text to learn probabilities of what word(s) comes next
S. Wolfram (2023) “What Is ChatGPT Doing … and Why Does It Work?”
Here’s the list.
progress sought | description |
---|---|
predicting values | predict an output value given some input parameters |
classifying data | classify some input parameters into one or more pre-determined classes |
discovering categories | discover categories within a given a set of data |
processing streams | “understand” a given input stream |
translating streams | turn an input stream into a output stream (that contains essentially the same information) |
generating outputs | generate a new output based on input. The output is typically a translation of an input stream, where the output contains different information than the input. |
We might identify that the progress sought explicitly talks in these terms. For example, I need to quickly classify medical images as containing cancer or not whilst my patient is with me. Or I need to translate from Swedish to English. And so on.
But sometimes we might need to dig s little into progress that implicitly relates to these categories. For example “I want to learn how to play the electric guitar in the comfort of my own home without the high cost of a guitar teacher”. Here we could identify that learning implies some form of testing. And that testing could relate to sampling my playing and comparing it against a reference. Letting me know where I was right and wrong in both tune and timing. This is an implicit example of AI processing streams.
Its therefore interesting to not just look at the generic examples, but to go further and look at some examples of each category.
Progress sought | Examples |
---|---|
predicting | linear, such as house prices Time-series – time dependent prediction Predictive maintenance / modelling Demand forecasting Recommendation Fraud detection Scheduling |
classifying | Binary – Yes/no Image/Video/Pattern recognition Anomoly detection Sentiment Analysis |
discovering clusters | Grouping, e.g. customer segmentation customer behaviour Detecting development delay in children |
processing/comparing streams | Natural language processing Examining learning (music playing, language…writing tests) Supporting learning (answering questions) Virtual Assistants Plagiarism detection |
translating streams | Language/speech translation Encoding |
generating | Chatbots Customer Service Automation Creating/Improving images / audio / text / voice / videos Summarising text/captioning images/video Expert/Engineer on shoulder |
The main constraint, at least as of 2023, is that AI is good at specific learn tasks. But I’d not generic AI. That is to say, it’s not capable of general learning outside specific task it trains upon. A customer service agent automation AI is not going to perform well looking for structural faults in steel.
And it’s not just functional progress. There are non-functional elements that AI can help with.
non functional progress sought
There’s a lot of non-functional Progress that AI can help with. These are in addition to the functional progress. If we review Almquist’s hierarchy of value (which we shrink a little and reprise as examples of non-functional progress) we can see this.
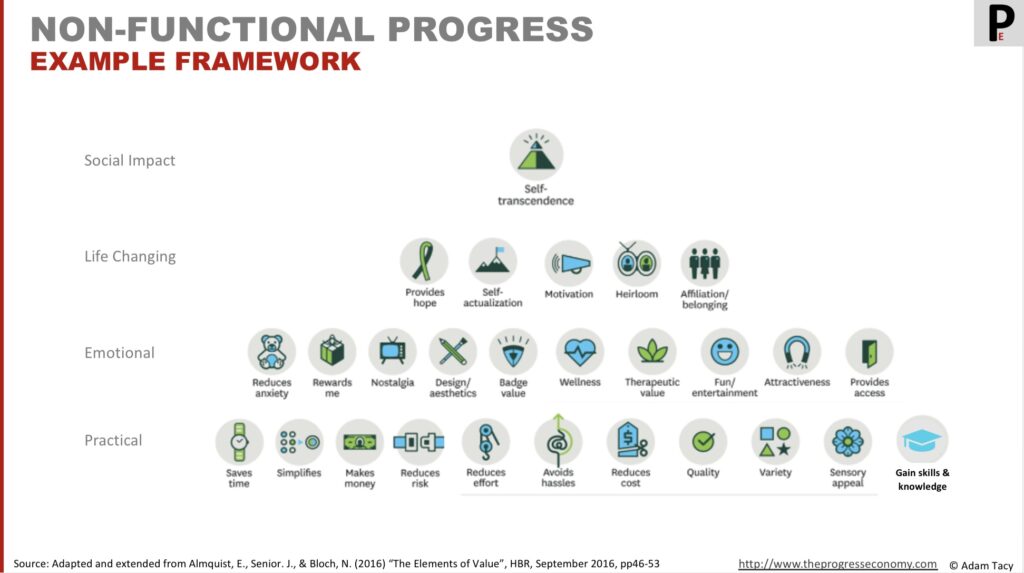
And it reaches quite high up the hierarchy.
I use a mobile app called Racefox to Practice cross country skiing. It takes info from motion sensors and identifies where I’m less than optimal in my technique. It translates that to a set of physical exercises and recommendations. With intention of improving my sling. It hits the following non-functional progress: motivates, wellness, rewards, reduces cost.
Education related AI helps with self actualisation and provides help as well as provides access. It might also be fun.
AI undoubtedly reduces effort.
Speed/availability might help answer the question why seekers begin to accept AI such as virtual assistants, customer service automation etc.
contextual progress sought
Context Progress explains to us the constraints in which progress is being sought. For example, travelling from A to B might have a constraint of doing so during rush hour traffic. That might narrow down viable offers of help.
A constraint AI helps manage is scalability. If you have 10 employees these typically can integrate with 10 seekers at a time. If you have 100 seekers, you need to find 90 more employees. With AI, such scalability is limited to how much hardware you have. And getting more hardware is relatively simpler than new employees. And with AI there are no training costs with these new “employees”. Similarly there are no costs if those “employees” are sitting idle. Nor if you need to downsize.
There is some translation needed to get from seeker expressed contextual progress – if they even explicitly express it – to scalability. We may find “no waiting time” or “instant access”. Or perhaps hiding in non-functional progress such as “quickly”.
Additionally, AI helps us break the “one integration” up over time. A seeker no longer needs to be integrating in a continuous period. They could, for example, start an integration in the morning, come back to it at lunch time, and compkete when they are at hone. Admittedly, this is more an attribute of moving to a system from employee. In a similar vein, contextual progress such as on demand fits here.
repeatedly, many examples
Affecting the progress hurdles
We know the progress economy informs us there are six progress hurdles with a progress proposition. Each need to be felt as low enough for a seeker to decide to attempt making progress with a proposition.
These hurdles are:
hurdle | description |
---|---|
lack of resource | is there (still, or newly introduced) a lack of resource that will hinder progress |
adoptability | can the progress seeker readily see themselves using the proposition (eg Rogers’ innovation adoption) |
resistance | will the progress seeker postpone, reject, or worse, oppose the proposition |
misalignment on continuum | how far apart, on the progress proposition continuum, are the proposition and the seeker’s wishes |
lack of confidence | does seeker trust proposition and/or helper |
effort elsewhere | how many service credits does a progress seeker need to get from elsewhere to engage proposition |
Lack of resource
Our first hurdle is lack of resources. That is to say the progress seeker lacks skills and knowledge to make the progress they are seeking.
AI is clearly aimed at minimising this hurdle. In a narrow area. Better still, AI democratises access to those skills and competence. Using Google translate is much more accessible than hiring a human translator. AI in education is helping more people learn more things.
And as we discussed earlier, AI is scalable, making encapsulate skills and knowledge more widely available.
But seekers may not understand how to integrate their resources with the AI. And that is an introduced lack of resources. However, it is interesting that this is creating opportunities for new progress propositions that offer to help seekers integrate. Check out the number of “I will help you with ChatGPT queries”, “I’ll build a chatbot for your site” and “I’ll create art for you with DALL-E” currently on offer at fiverr.com.
This is very much in line with what the progress economy thinking tells us. That is to say, a number of seekers who acquire new skills and competence will then offer those as progress propositions to others in a desire for service exchange.
Adoptabilty
There seems no lack of appetite to adopt this current AI summer of deep learning tools.
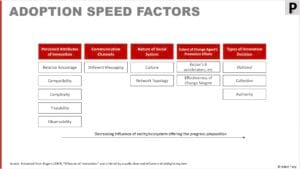
This is likely due to the cool and exciting nature of them. And that they offer a strong relative advantage – in most cases the alternative is to do nothing. Additionally, observability and trialability appear high. Particularly with public facing generative tools. Such as ChatGPT and various image generation tools
Complexity of use should be low. Especially with the use of natural language interfaces. And as we see above, where tools may be seen as complicated, a growing set of “I can help you use tool X” progress propositions can flourish.
Resistance
For sure there will be resistance to the use of AI tools. And Kleijnen et al framework helps us explore that.
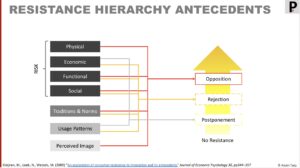
First there is how will seekers view using AI instead of employees in a mix. That is to say where AI has automated employees. How far does that go against traditions & norms leading to opposition and/or rejection? I suspect this is largely contextual on societal impact.
Proposing to make teachers redundant being more meaningful than automating some of their tasks. Using AI that could, through failure, damage human life will challenge resistance. We’re close to having self-driving cars…
Concerns of deep fakes and biases. Going wrong, eg ms tay https://www.huffpost.com/entry/microsoft-tay-racist-tweets_n_56f3e678e4b04c4c37615502
https://www.nytimes.com/2023/02/16/technology/bing-chatbot-transcript.html
/techmonitor.ai/technology/ai-and-automation/will-2023-be-year-of-ai-lawsuit
Alignment on proposition continuum
AI tools can be seen as sitting at various positions on the progress proposition continuum.
Confidence
Google bard: https://www.forbes.com/sites/jonathanponciano/2023/02/08/alphabet-google-stock-plunge-erases-100-billion-after-new-ai-chatbot-gives-wrong-answer-in-ad/
www.nist.gov/itl/ai-risk-management-framework
Effort elsewhere
Value co-creation and co-destruction
https://www.frontiersin.org/articles/10.3389/fpsyg.2022.863313/full
www.bbc.com/news/business-64371426
Wrapping up
In fact, this whole article was written by AI! OK, only joking. But with the availability of tools such as ChatGPT many articles elsewhere might well be. I wrote this myself, but artificial intelligence has helped suggest how to make the text flow smoother (Quillbit). And it could create a video of this article complete with lip synced avatar (e.g Synthesia). AI is the artist behind the cover image, creating art from some text that I provided, addressing my lack of drawing skills.
Let’s progress together through discussion…